Category: Best Practices
Posted by: bagheljas
Industry Leading Approach is to create an Enterprise Data Economy built on Data Collaboration, Data Democratization, and improved User Experiences for Data Research and Artificial Intelligence. It has ingrained Data Privacy, Security, and Resiliency capabilities. The solution design uses the following guiding principles those are from the article, Data Platform Innovation: Industry Leading Practices.
Use Reference Architecture from the article, Data Platform Buzzwords: Introduction and So What?, to assess and design the Data Platform Target State Architecture.
- Cloud First while extracting the life of current IT investments
- Utilize Data Ops to automation for Data Services and Backup & Restore Data
- Utilize Distributed Data Architecture and Data Mesh for Data Democratization
- Design for real-time use cases and ease of data research tools integration
- Flexible Data Stores with anytime availability of the Raw Data
- Identify and Use AI Tools to Handle Data Quality Issues
- Utilize Data Hub, Data Fabric, and Data Navigation for ease of Data Discovery and Collaboration
Use Reference Architecture from the article, Data Platform Buzzwords: Introduction and So What?, to assess and design the Data Platform Target State Architecture.
Category: Best Practices
Posted by: bagheljas
Availability and Applications of Data have emerged as a business innovation engine of the present time and for the foreseeable future.
Data Platforms are a conglomerate of Business Requirements, Architectures, Tools & Technologies, Frameworks, and Processes to provide Data Services. Hence, the Data Platform Innovation foundation is from people, processes, and technology managing and utilizing an enterprise Data Platform. In the article, I have organized the emerging Industry Leading Practices into seven Pillars to maximize data value at speed in an enterprise environment.
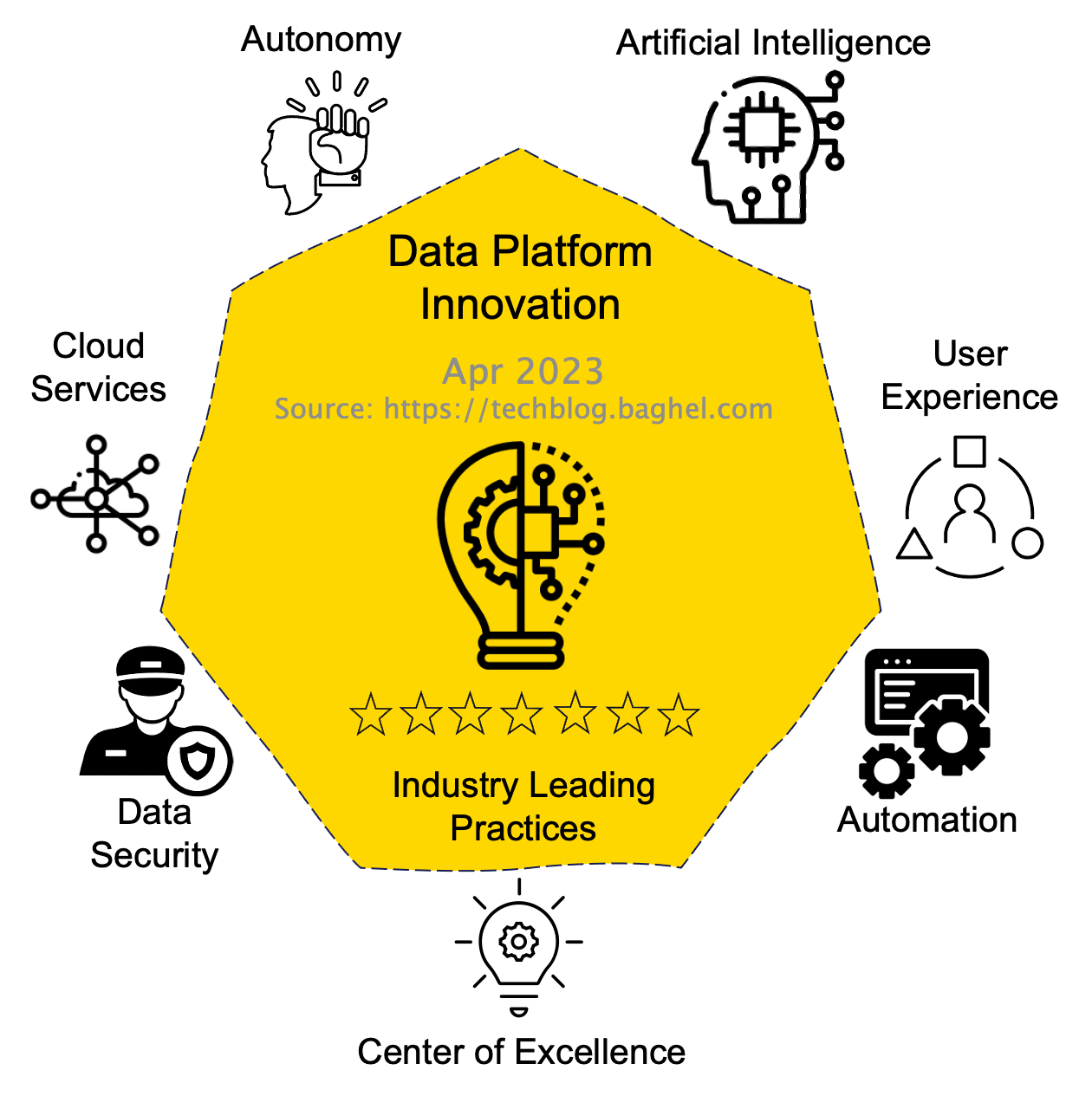
Pillars - Data Platform Innovation: Industry Leading Practices
Data Platforms are a conglomerate of Business Requirements, Architectures, Tools & Technologies, Frameworks, and Processes to provide Data Services. Hence, the Data Platform Innovation foundation is from people, processes, and technology managing and utilizing an enterprise Data Platform. In the article, I have organized the emerging Industry Leading Practices into seven Pillars to maximize data value at speed in an enterprise environment.
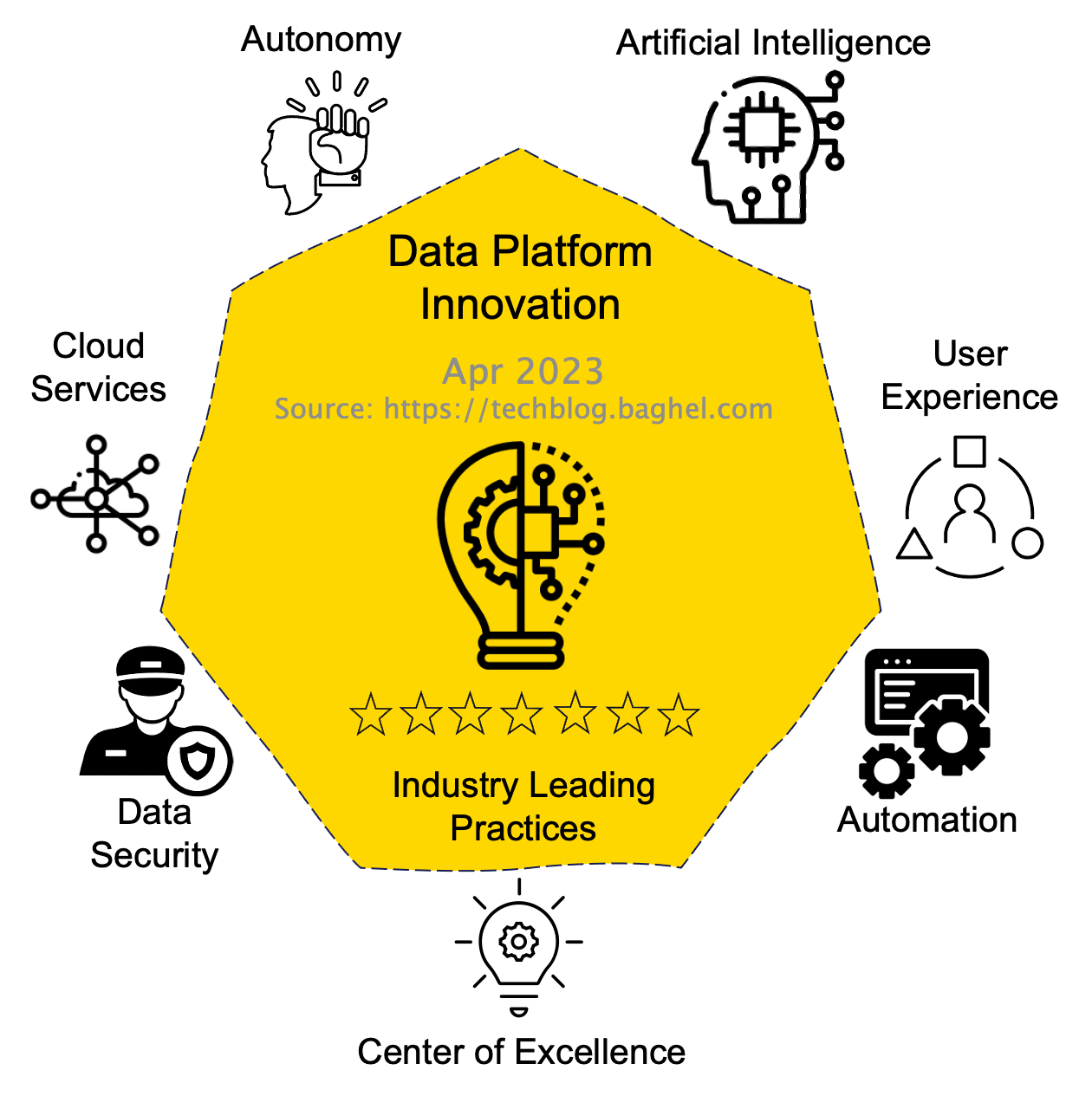
Pillars - Data Platform Innovation: Industry Leading Practices
- Autonomy
- Implement Data as a Product with an operating model that establishes data product owner and team.
- Support Data Democratization utilizing Distributed Data Architecture and Data Mesh.
- Enable end-to-end service delivery ownership to the Data product owner.
- Artificial Intelligence (AI)
- Create a raw Data copy availability to enable AI Data Models yet to be discovered.
- Utilize AI Tools to manage Data identification, correction, and remediation of Data quality issues.
- User Experience
- Create and manage data literacy and data-driven cultural activities for employees to learn and embrace the value of data.
- Enable data navigation and data research tools for employees.
- Automation
- Utilize DataOps at the heart of provisioning, processing, and information management to deliver real-time use cases.
- Implement automatic backup and restoration of Data and digital twins of the Data estate.
- Center of Excellence
- Shift from stakeholders' buy-in approach to delivery partners' approach that finds and enables innovation.
- Create Data Eco-System utilizing Data Alliances, Data Sharing Agreements, and Data Marketplace to develop an Enterprise Data Economy.
- Publish Common Data Models, Policies, and Processes to promote ease of collaboration within and across organizations.
- Data Security
- Contribute actively to individual data-protection awareness and rights.
- Communicate the importance of data security throughout the organization.
- Develop Data privacy, Data ethics, and Data security as areas of competency, not just to comply with mandates.
- Cloud Services
- Cloud First mindset for quickly exploring and adopting innovation at speed with minimal sunk cost once that becomes mainstream. Let the business model drive the Cloud equilibrium.
- Enable cloud for flexible data model tools supporting querying for unstructured data.
- Enable edge devices and high-performance computing available at Data sources to deliver real-time use cases.
Shortly after earning a Master of Science in Operations Research and Statistics at the Indian Institute of Technology (IIT Bombay), I joined as a Research Scientist in the Artificial Intelligence Lab for the Computer Science Department. I developed an AI application for routing and scheduling crews that delivered operation efficiency of 36% for our customers and won numerous awards and national recognition.
Later, moving to the United States, I worked as a Software Engineer with companies such as AT&T and IBM. One key highlight was developing security architecture for single sign-on for over 200 mission-critical apps at AT&T. It was followed by a stint with NLM, where I delivered Data Integration between the NLM and FDA, Search Engine, and Conversational AI for self-service.
Next, I worked at Aol as an Operations Architect, a key role in enabling billing and subscription services and setting up enterprise standards and best practices. While at Aol, I earned a Master of Science in Technology Management at George Mason University and a Chief Information Officer Certificate at United States Federal CIO University.
Over the last ten years, I have led consulting, solution center, and pre-sales at SRA International / General Dynamics Information Technology, CenturyLink / Savvis / Lumen, and IBM / Kyndryl. Notable Accomplishments in Apps and Data Space:
I have co-founded:
Learn more about me:
Later, moving to the United States, I worked as a Software Engineer with companies such as AT&T and IBM. One key highlight was developing security architecture for single sign-on for over 200 mission-critical apps at AT&T. It was followed by a stint with NLM, where I delivered Data Integration between the NLM and FDA, Search Engine, and Conversational AI for self-service.
Next, I worked at Aol as an Operations Architect, a key role in enabling billing and subscription services and setting up enterprise standards and best practices. While at Aol, I earned a Master of Science in Technology Management at George Mason University and a Chief Information Officer Certificate at United States Federal CIO University.
Over the last ten years, I have led consulting, solution center, and pre-sales at SRA International / General Dynamics Information Technology, CenturyLink / Savvis / Lumen, and IBM / Kyndryl. Notable Accomplishments in Apps and Data Space:
- SRA International / General Dynamics Information Technology: Executed the modernization of the Centers for Disease Control and Prevention's vaccine adverse event reporting system (The Largest Program in the World) to enable self-service and green initiatives to deliver digital reporting and remote work options.
- IBM / Kyndryl: Onboarded a large new logo client in the insurance industry to adopt Hybrid Cloud, DevOps, and API-driven managed services to deliver on-demand one-click Guidewire Apps environments, reducing the environment delivery timeline from 45 days to 8 hours.
I have co-founded:
Learn more about me:
Modern Enterprise Data Platforms are a conglomerate of Business Requirements, Architectures, Tools & Technologies, Frameworks, and Processes to provide Data Services.
Data Platforms are mission-critical to an enterprise, irrespective of size and industry sector. Data Platform is the foundation for Business Intelligence & Artificial Intelligence to deliver sustainable competitive advantage for business operations excellence and innovation.
Data Platforms are mission-critical to an enterprise, irrespective of size and industry sector. Data Platform is the foundation for Business Intelligence & Artificial Intelligence to deliver sustainable competitive advantage for business operations excellence and innovation.
- Data Store is a repository for persistently storing and managing collections of structured & unstructured data, files, and emails. Data Warehouse, Data Lake, and Data Lakehouse with Data Navigator are the specialized Data Stores implementations to deliver Modern Enterprise Data Platforms. Data Deduplication is a technique for eliminating duplicate copies of repeating data. Successful implementations of Data Deduplication optimize storage & network capacity needs of Data Stores is critical in managing the cost and performance of Modern Enterprise Data Platforms.
- Data Warehouse enables the categorization and integration of enterprise structured data from heterogeneous sources for future use. The operational schemas are prebuilt for each relevant business requirement, typically following the ETL (Extract-Transform-Load) process in a Data Pipeline. Enterprise Data Warehouse Operational Schemas updates could be challenging and expensive; Serves best for Data Analytics & Business Intelligence but are limited to particular problem-solving.
- Data Lake is an extensive centralized data store that hosts the collection of semi-structured & unstructured raw data. Data Lakes enable comprehensive analysis of big and small data from a single location. Data is extracted, loaded, and transformed (ELT) at the moment when it is necessary for analysis purposes. Data Lake makes historical data in its original form available to researchers for operations excellence and innovation anytime. Data Lakes integration for Business Intelligence & Data Analytics could be complex; best for Machine Learning and Artificial Intelligence tasks.
- Data Lakehouse combines the best elements of Data Lakes & Data Warehouses. Data Lakehouse provides Data Storage architecture for organized, semi-structured, and unstructured data in a single location. Data Lakehouse delivers Data Storage services for Business Intelligence, Data Analytics, Machine Learning, and Artificial Intelligence tasks in a single platform.
- Data Mart is a subset of a Data Warehouse usually focused on a particular line of business, department, or subject area. Data Marts make specific data available to a defined group of users, which allows those users to quickly access critical insights without learning and exposing the Enterprise Data Warehouse.
- Data Mesh:: The Enterprise Data Platform hosts data in a centralized location using Data Stores such as Data Lake, Data Warehouse, and Data LakeHouse by a specialized enterprise data team. The monolithic Data Centralization approach slows down adoption and innovation. Data Mesh is a sociotechnical approach for building distributed data architecture leveraging Business Data Domain that provides Autonomy to the line of business. Data Mesh enables cloud-native architectures to deliver data services for business agility and innovation at cloud speed. Data Mesh is emerging as a mission-critical data architecture approach for enterprises in the era of Artificial Intelligence. The Data Mesh adoption enables the Enterprise Journey to Data Democratization.
- Data Pipeline is a method that ingests raw data into Data Store such as Data Lake, Data Warehouse, or Data Lakehouse for analysis from various Enterprise Data Sources. There are two types of Data pipelines; first batch processing and second streaming data. The Data Pipeline Architecture core step consists of Data Ingestion, Data Transformation (sometimes optional), and Data Store.
- Data Fabric is an architecture and set of data services that provide consistent capabilities that standardize data management practices and practicalities across the on-premises, cloud, and edge devices.
- Data Ops is a specialized Dev Ops / Dev Sec Ops that demands collaboration among Dev Ops teams with data engineers & scientists for improving the communication, integration, and automation of data flows between data managers and data consumers across an enterprise. Data Ops is emerging as a mission-critical methodology for enterprises in the era of business agility.
- Data Security needs no introduction. Enterprise Data Platforms must address the Availability and Recoverability of the Data Services for Authorized Users only. Data Security Architecture establishes and governs the Data Storage, Data Vault, Data Availability, Data Access, Data Masking, Data Archive, Data Recovery, and Data Transport policies that comply with Industry and Enterprise mandates.
- Data Hub architecture enables data sharing by connecting producers with consumers at a central data repository with spokes that radiate to data producers and consumers. Data Hub promotes ease of discovery and integration to consume Data Services.
Disclaimer
The views expressed in the blog are those of the author and do not represent necessarily the official policy or position of any other agency, organization, employer, or company. Assumptions made in the study are not reflective of the stand of any entity other than the author. Since we are critically-thinking human beings, these views are always subject to change, revision, and rethinking without notice. While reasonable efforts have been made to obtain accurate information, the author makes no warranty, expressed or implied, as to its accuracy.
The views expressed in the blog are those of the author and do not represent necessarily the official policy or position of any other agency, organization, employer, or company. Assumptions made in the study are not reflective of the stand of any entity other than the author. Since we are critically-thinking human beings, these views are always subject to change, revision, and rethinking without notice. While reasonable efforts have been made to obtain accurate information, the author makes no warranty, expressed or implied, as to its accuracy.